Alternatives to (math) academia and how to get there
[This is a reference post aimed at mathematicians (PhDs, postdocs, professors) who might be interested in options outside of academia. It outlines common career paths and how to find a foothold in those directions. This might be of some limited use to those who are interested in the careers but come from a different background. This is unlikely to be of use to people with neither the background nor the career interest.]
I've had this conversation a bunch of times now, and I'm happy to talk about it each time, but it seems like it would be more convenient for all parties if there were just a compiled list of my advice somewhere, so here's an attempt at such a compilation. Apologies in advance for any gaps, please feel free to email me with questions, and answers may get added to this reference, though I think I'm approximately aiming for an 80/20 rule here.
Why leave?
Whatever reasons there are will be highly personal. I can list some of my own reasons below, as well as some that I've heard, but if you're reading this, you might have some of your own as well. Note that this is unashamedly biased towards leaving academia, but I don't think someone is wrong if they conclude that staying in academia is right for them, so long as the decision is well-informed.
But my first lukewarm take is that the discoverability of other options is generally terrible, and the friction of figuring out what options there are and how to actually achieve those options is enough to stop many people from seriously considering an exit.
I'll also note a personal mental obstacle that I had as a young grad student. For whatever reason, I had a sort of cached mental impression that not "making it" to an academic job was a failure. It's hard to pinpoint exactly where that impression came from. I'm sure some of it was internal, but there's also a degree to which this view is baked in around us – for example, it's common to refer to leaving academia as selling out, which has some negative connotation. I don't think holding the view that it's a failure is healthy, nor is it true.
In reality, I think most of the reasons why someone might remain in academia are superseded by the appropriate arrangement outside of academia (of which there are many), up to and including being able to do more research outside of academia. Read on for more details.
Reasons to leave
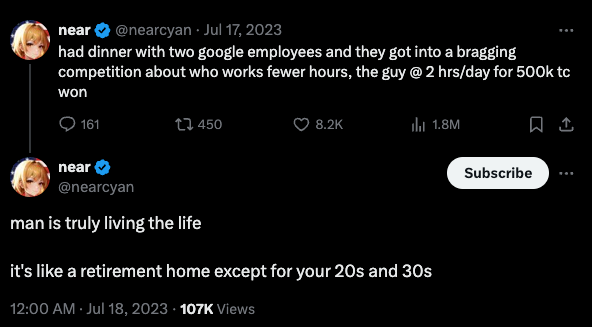
- You make (a lot) more money outside of academia
- By the time you would get tenure in academia, you could counterfactually be a senior or staff level researcher/engineer (see later on for a note on "levels") in tech and earn 300-500k+ total compensation (combined salary + equity grant, which is as good as cash)
- The opportunity cost of staying in academia is well into the six figures per year, just from the raw salary difference, not even accounting for compounding growth of investments. If you weren't already in academia would you pay $200k+ per year in order to be in academia? (if you look further down, my estimation of the opportunity cost at the tenured professor level is much higher)
- The job demands, stress, and time commitment are typically lower
- The demands are often low enough that you can hold a full time job and still have more time for research than in academia
- The Google employees in the tweet above are probably on the low end, but not that far from the true median.
- You have more flexibility in where you live (especially with remote work)
- Remote work in tech specifically also comes with extremely flexible hours
- Less administrative burden, zero course preps, no outreach/service expectations
- If you learn certain practical skills, you are incredibly employable and don't need the job security guarantees of tenure (and make enough money anyways that you can absorb unlikely periods of unemployment, or just straight up retire after ~10-20 relatively chill years and just do whatever the hell you want after)
- There may be a personal situation tying you to your job. Depending on that situation (e.g. with visa status), you may find a solution anyways outside of industry (many tech companies sponsor visas)
Where do I go?
Ok, so let's say you're considering leaving. What are your career options? When I first Googled "math major career options" in undergrad, the top result was being an actuary. Nothing against actuaries, they're probably cool people, but I don't want to personally find out. Your career options are much more varied than this.
- Tech / Silicon Valley
- This can mean researcher (e.g. machine learning, data science, etc) or software engineer
- This is broadly split into Big Tech (FAANG companies) and startups
- Big Tech has the highest compensation between the two and the lowest job demand (which is paradoxical, but FAANG makes a lot of money, and it's hard to be ultra efficient at their scale)
- As an intuition pump: Elon caught a lot of flak for firing like, 80% of X (f.k.a. Twitter), but it's still running (albeit with some performance degradation here and there). I was among the doubters, but so far it seems like he was right on this point. Many big tech companies could probably survive >50% cuts if they had the guts to do it.
- Startups have the most exciting work, but lower compensation (higher than academia) and higher job demand (comparable to academia). If you're an early employee at a startup that eventually IPOs, you're probably going to walk away very rich, but don't count on it. The attraction here is working on problems that feel like they're either building the future or really fixing existing problems in a meaningful way. Be careful not to choose a startup whose mission you don't care about. The entire point of working at a small startup is mission alignment. Working on a mission you do care about feels amazing. Some real examples of startups that you can really work on:
- Flying houses (mission: tiny houses that can be airlifted into disaster relief areas)
- Lab grown meat (mission: end animal suffering due to factory farming)
- Alternative energy (mission: solve world energy supply)
- Wearable tech (biometrics: live healthier lives / AI assistants: extend our cognition with new tools)
- Self-driving cars (lots of potential missions, e.g. solve car accident mortality)
- Literal space travel (mission: go to SPACE and maybe COLONIZE MARS)
- Those are just the eye-catching ones. If you can think of a real problem in the world, even if it's incredibly unsexy, there's probably a startup somewhere working on it (lots of startups also suck though and are working on fake problems, but evaluating startups is an entire 5k word guide on its own)
- Quant finance
- Pay is similar to big tech
- Great if you are specifically extremely competitive, resilient to stress, and can be happy with high variance positive EV decision making even if the realized consequence was negative (think activities like poker)
- There is a particular type of person for whom this is a good fit, but most people are not, and that is fine
- NSA
- The NSA employs a lot of mathematicians, but I'm not familiar with the precise kind of work they do. Presumably a lot of cryptography related work.
- I hear the work-life balance is great at the NSA
- CCR campuses
- NASA
- You can do cool space stuff!
- Government labs
- There are a variety of national labs that fund fundamental research
- If such a lab happens to fund the type of research you do, you can enjoy what is essentially an academic job but without the teaching
- Healthcare / biostats
- A close friend of mine does work in epidemiology using statistics and gets to do covid modeling
- Honestly, almost anything
- Your most powerful tool is being agentic. You can, in some sense, really do whatever you put your mind to – most people are limited by artificial walls. A PhD is proof that you can do hard stuff on your own. The degree of career freedom you have outside of academia is incredible. Not all artificial walls are worth tearing down, but before you think "I could never do that," look with fresh eyes and ask if that's really true.
- There are plenty of careers that there aren't degrees for. America employs ~160M people, and my undergrad institution had like ~120 majors. Lots of people have jobs that they didn't formally study for, and you can do that too.
- Things that I did or almost did at various points (in the span of only 2.5 years!) that no one had to give me permission or a formal list of prereqs for: education tools, music tech, science communication, game design, sports betting (for American football – and I still don't even know what a tight end is), algorithmic trading, software engineering, blockchain/web3, tech consulting/advising for early startups, data science, supply chain optimization, AI safety research, and probably a lot more that I've forgotten already
- Other thoughts
- Perspectives that people have on PhDs are mixed, but there's more than enough people who look at it and say "oh shit, they have a PhD, they're probably really smart" rather than "their thesis isn't relevant, let's not hire them" that I think it's net positive (you only need one good hiring manager to think positively towards a PhD)
- There is a ton of interesting work that happens outside of academia.
How do I actually, concretely get a job?
[This is from the perspective of a student, but postdocs and professors can adapt the same general strategies.]
For most of the common career paths, the primary new skill you need to pick up is some degree of programming (coding combinatorics experiments is a good starting point, but not representative of the skills you need) and/or some degree of statistics/data science/machine learning (these three all blend together to different degrees at different jobs). Everything else you can learn on the job (and don't worry – everyone does). If you're picking the "choose-your-own-adventure" option in the previous section, then I'll leave it up to you to determine how much of what skill you need to learn and how you learn it.
- You probably don't need to learn much programming, if any, for jobs like at the NSA or at government labs (or if you do, they might just teach you on the job)
- You need to learn a little programming (probably leaning towards statistical languages, e.g. Python, R) but mostly data science concepts for things like biostats and data science
- You need to learn a decent amount of programming if you want to do software engineering
- You need both programming (less than for software engineering) and data science/ML for quant finance
- You need both if you want to specialize to certain parts of software engineering, such as machine learning engineering
- Lukewarm take, but I think basically any job in the realm of knowledge work will benefit from some proper programming experience, even if the job description doesn't explicitly call for it (and even if none of your coworkers know any)
From here on, the main focus will be on getting tech/quant/data science jobs. The reason for this is that these are 1) what I'm most familiar with and 2) what I expect will be the most involved processes / require the most domain specific prep knowledge.
Learning data science and machine learning
Your core resource is Kaggle. Start with the Titanic and Housing Prices intro competitions (in that order). Find some example notebooks, or if you're braver, try to analyze the dataset and generate predictions on your own without hints. These notebooks exist to familiarize you with the common tools, languages, and techniques that are used in data science. Pay attention to the questions asked, but also the heuristics and rigor. Notice the types of questions which are not asked. Data science is a different field from mathematics with different standards for what is or isn't a good answer to a question.
Work your way up to trying some live competitions, including competitions with real prize money. Pick one that seems interesting, maybe find a few friends who are also trying to learn data science and form a team. Give yourself somewhere from a few weeks to a couple months and dig really deep into that competition. There is no substitute to learning by doing.
As a reference point for the level of difficulty of questions you might get in interviews:
- One of my onsite questions at a quant hedge fund was essentially a series of questions about the housing prices dataset
- Another ML/data science role asked me to code the k-nearest neighbors algorithm from scratch (I later learned that they didn't mean literally from scratch, it was meant to be a test of how well I knew the numpy library in Python)
- Various questions on linear regression. These types of questions did not inherently require knowledge beyond the undergraduate level, but they did require a depth of understanding of those concepts that wouldn't normally be expected of an undergraduate.
- E.g. suppose you have a dataset on the 2D plane, where data is uniformly distributed in a rectangle. What happens to the linear regression line as you rotate that rectangle 90 degrees clockwise or counter-clockwise?
- Similar questions about normal distributions are common
Learning to program
Programming experience in the software industry is typically split into "production" and "not production" level experience. "Production" means work that was done in a commercial setting or otherwise at a commercial level. This typically implies things such as: real money is on the line, code is run live without supervision most of the time, and coding is done as a team in a shared repository with peer reviews, among other things. Unfortunately, within the software industry, things like personal projects or coding experiments for math research are not typically considered production-level coding experience.
At first glance, there is an apparent catch-22 that it's hard to find a job if you don't have production-level coding experience, but you can't get production-level coding experience if you never get a job. Internships can be one solution, but maybe you're applying in your last year and you don't have any summers left, or maybe you're a postdoc/professor already. In that case, here is a "minimum viable solution" to getting out of the catch-22:
- Watch the MIT OCW Introduction to Algorithms videos. Doing problem sets is optional.
- Become comfortable with the data structures and algorithms presented as well as in the analysis of time and space complexity of solutions.
- Doing problem sets in the previous step is optional because doing problems in this step is not optional. Make a LeetCode account, consider if a premium subscription is useful to you (I bought one month of premium at one point and found it useful enough for one month but not more), and then do a ton of problems.
- Start with easy problems, then move up to medium once you feel good about easy problems.
- There is a (now somewhat infamous maybe) list of 75 LeetCode problems that aim to be representative of the types of problems you'll run into in interviews. If you're pressed for time (e.g. graduating soon), this is the minimal set of LC problems you should aim to solve
- LeetCode is seriously the core of the current meta of (entry level) tech interviews. It is a fake skill in the sense that the connection with ability to do real work is dubious (though I don't think it's totally indefensible), but it is a real skill in the sense that you can really study to the exam and get good at LC style problems and this is highly instrumental for getting a job in the first place.
- A good benchmark is being able to consistently do LeetCode medium problems on your own, with no hints, in 15-30 minutes. The vast majority of interview problems are not harder than LeetCode medium. Past this point, you start to get diminishing returns on the time spent on LeetCode, but it is (IMO) quite hard to accidentally get to a point where marginal practice is pointless.
- Quick plug for a fun alternative to LeetCode: Advent of Code. This is an annual advent calendar of coding problems that increases in difficulty as it approaches Christmas and has a silly story to go along with it.
- Finally, work on some actual personal projects. Find something interesting and just do it.
- You can sometimes get a 2 for 1 with data science/ML experience by doing some kind of coding-intensive competition on Kaggle.
- The first project I ever worked on that made it onto my resume was an automated arbitrage bot for Magic The Gathering: Online. The code was trash, it was a buggy mess (in our defense, this was partly because MTGO itself was a buggy mess), and we got banned from the platform shortly after. We made some actual money off of running the bot, but the real value was returns on career capital – it has one of the highest return rates of any single week I've ever spent coding. It is the most common talking point in my interviews and is still proudly displayed on my resume.
- Check out this Hacker News thread for examples of long-tail outcomes of interesting projects
- Another avenue to show off some of your coding (and to actually get some real code review/feedback) is to make open source contributions on Github. I won't get into how to do this, but some employers view this very favorably and like to see actual code examples as a way to de-risk hiring you.
- A longer path (which you can take if you decide well in advance that this is the path you'd like to take) is to take some computer science classes, and maybe even get a masters in CS. This seems effective if you're willing to put in the time commitment and can plan ahead for it.
Misc quant questions
There is a small industry built around certain types of quantitative problems and brain teasers that get asked almost exclusively in quant interviews. Getting into these problems is probably out of scope for this document, but you can find plenty of examples online.
General interview advice
Interviewing is a skill, too. Your first few interviews will probably feel like garbage. If I haven't interviewed for a while, my interview skills get rusty, and it takes a few warm-up interviews to get comfortable with them again. Bias towards interviewing earlier in your interview prep process and just get the crappy first few interviews out of the way. I promise it gets better, and there are enough companies out there that flubbing a few interviews for middle-range targets isn't an issue.
For most of the quantitative jobs above, the vast, vast majority of your interviews will be technical interviews. Other interviews (such as with HR or recruiters) will be more like formalities in the process, mostly just checking that you're reasonably easy to talk to. I didn't have a single real behavioral interview until one point where I was speculatively interviewing for a totally different kind of job.
For technical interviews, a full guide is also out of scope for this reference, but here are some general pointers:
- Think out loud (seriously, it is sometimes a red flag for interviewers if you are too quiet)
- Ask clarifying questions (green flag if you ask them, red flag if you don't + were wrong about interpreting the question)
- Don't be afraid to start with naive solutions to programming problems where the time complexity sucks and is obviously not optimal. As long as you say that out loud and then iterate from there, it's totally fine and counts as partial progress towards answering the question
- If a question seems really unusually hard, it might be your unlucky day, but it might also be the case that it's hard for everyone. Many places rate interview performance on problems "on a curve"
- For some questions, you can get more "points" by sharing high quality thinking out loud without arriving at the right answer than by just jumping to the right answer. What this looks like is: asking the right questions, thinking about the right things, coming up with tractable, reasonable sounding ideas (that may not ultimately work)
- If you know someone who is in tech, call in some favors and do practice interview sessions and get feedback
- The interview process can be pretty long; I think my longest interview processes had 5 rounds (where the last one is an "onsite," though these are often over Zoom now instead of physically onsite), and I've heard of more. Have a constant pipeline of interviews, and don't wait for one interview process to end before looking for another.
- Don't get too attached to any one job. I made this mistake a few times, and still ultimately ended up with a job I was very happy to get each time (and which was likely better than the counterfactual offer anyways)
A note on "levels"
One of the great (IMO) features of tech careers is the notion of a "level." Although they aren't fully standardized between different companies (and it takes some experience to know what to expect from a "senior" engineer coming from different types of orgs), I still find them a pretty useful framework for understanding jobs in tech. A typical sequence of levels at tech companies (or quant sometimes, for larger firms) looks something like the following, where if you're on say, a data scientist track, you might replace "software engineer" with "data scientist" everywhere.
- Junior
- Sometimes can be a title like "Software Engineer 1" or "SDE 1" or "SWE 1"
- Entry level for bachelors
- Very low expectations, your job at this level is mostly to learn. Companies typically view juniors as an investment more than a clear source of value.
- Mid
- Often comes without a title prefix, e.g. just "Software Engineer" and not "Mid-level Software Engineer"
- Otherwise might look like "Software Engineer 2" or "SDE 2" or "SWE 2"
- Senior
- Typically considered a "terminal" role in tech – there are many levels beyond this, but this is where you're considered good enough for companies to be happy to have you sit here indefinitely and provide this level of value without growing further. Many people do just that.
- Typical expected time is ~5 yrs to reach this level (for the median engineer that makes it to senior level, not for the median engineer with a PhD that makes it to senior – you can make it to senior much faster if you want but you do not have to)
- When interviewing for this level and above in software, you will start to need to know system design. Expect at least one technical interview to be on system design problems.
- Staff
- This is where there's an option to move into the management track, which has its own levels that parallel the "individual contributor" (IC) track
- Most people actually move into management instead of moving up the IC ladder, see notes on that below
- It is harder to define what your role is at this level and above, staff+ ICs tend to get increasingly specialized roles based on their individual capabilities and the needs of the company
- Compensation was already stupid, but this is where it gets real dumb. 500k+ total compensation is common at FAANG
- This is where there's an option to move into the management track, which has its own levels that parallel the "individual contributor" (IC) track
- Principal
- Here levels start to break down further at different companies. Sometimes there is a "senior staff" before this level and sometimes there is a "senior principal" after this level. Sometimes staff is skipped, sometimes principal doesn't exist.
- Compensation gets downright offensive: FAANG principal+ can earn 7 figures
Management track has similar levels (and compensation) starting with "Engineering Manager" or something similar being the equivalent of something like "Staff Software Engineer." It then has its own titles like "Senior Engineering Manager," and may go towards titles like "VP of Engineering." All of these levels (for both IC and manager track) have their own expectations, which I won't cover here. There are good/comprehensive resources online, like this one.
Benchmarking levels against academia
Ok, here's where the real hot takes begin. I'm not going to bother justifying these, so take them with a grain of salt, but I think this is what I believe from my limited perspective.
- If you don't have a PhD in computer science or machine learning, expect to enter tech or quant at the junior or mid-level. You might be able to get a data science role at a senior level if you did e.g. probability and do well on your interviews.
- The startup I first worked for didn't have levels in a formal sense until a year after I joined, but I think I was retroactively initially placed at the junior level and then promoted to mid-level after a year.
- If you can get a PhD in math, you can reach senior level in tech, full stop. Tech jobs are high paying because 1) tech companies make a shitload of money, and marginal engineers really do actually bring a lot of value to the company at scale and 2) because the jobs are competitive and hard and have high expectations. But crucially, they are competitive and hard and have high expectations relative to the typical job in America. They are not more competitive or harder or have higher expectations than earning a PhD (modulo personal fit for tech, some people may just find software easier than others).
- Postdocs up through tenured professors are probably somewhere around the "staff" level equivalent for academia.
- I'm less sure about this, but I'd guess that full professor in academia is comparable to principal level in tech or to the equivalent management track role (hey, remember that 7 figure compensation number above?)
- These don't mean they're where your cap is, but I would confidently place money at 1:1 odds (and probably much worse odds, though I don't want to commit to figure those out here) that someone with the given level of academic accomplishment can first reach the given comparable tech level in a typical amount of time
- In general, the number of people who reach each progressive level in the tech ladder tends to decrease exponentially, and the difficulty and expectations of the levels also increase substantially past senior. It is totally fine to hit ~senior level and just chill there forever and do other stuff with your time, like fun side projects or your math research or just having more time to enjoy life in general.
A personal request
If you've gotten this far, hopefully this resource has been useful to you. I am happy if this improves your life in any way. My only request to you is that you please try to avoid working directly on AI capabilities as a result of this advice.
I've written about AI safety previously and have pivoted my career to technical safety research because I think this is such an important problem. This is not asking you to say, turn down the only offer you get and not be able to support yourself or your family, but to please think about this if you find yourself having a choice in the matter. Most job opportunities don't fall under this umbrella, and it'll probably be clear if a given job is aimed at significantly advancing AI, so you mostly have to be specifically looking for such jobs.